Biorobotics Lab
Wearable soft sensor and DNN-based signal processing for joint angle estimation
A wearable soft sensor has been utilized to measure a human body motion, but its inherent nonlinearity and hysteresis are regarded as major drawbacks. The sensing performance of the sensor can be degraded by its nonlinearity and hysteresis. Thus, these characteristics have to be attunuated for more accurate estimation.
Our researchers have focused on a low-hysteresis stretch sensor and a deep neural network (DNN)-based signal processing. Since the capacitive-type soft stretch sensor has shown a high linearity and repeatability, we deployed multiple capacitive-type soft stretch sensor on the ankle to obtain the body motion data. Even though the sensor makes reliable and accurate output, the it is still challengin to convert the sensor output into the joint angle due to the human kinematics. Therefore, we developed the joint angle estimation algorithm by using DNN with a transfer learning. We trained the DNN model with a simulation data instead of the experiment data. This DNN model can be used to convert the sensor data into the joint angle, but the modelling error makes some error. Hence, we also calibrate the trained model by using a small number of experiment data. As a result, the human body motion can be accurately estimated by the soft sensor suit.
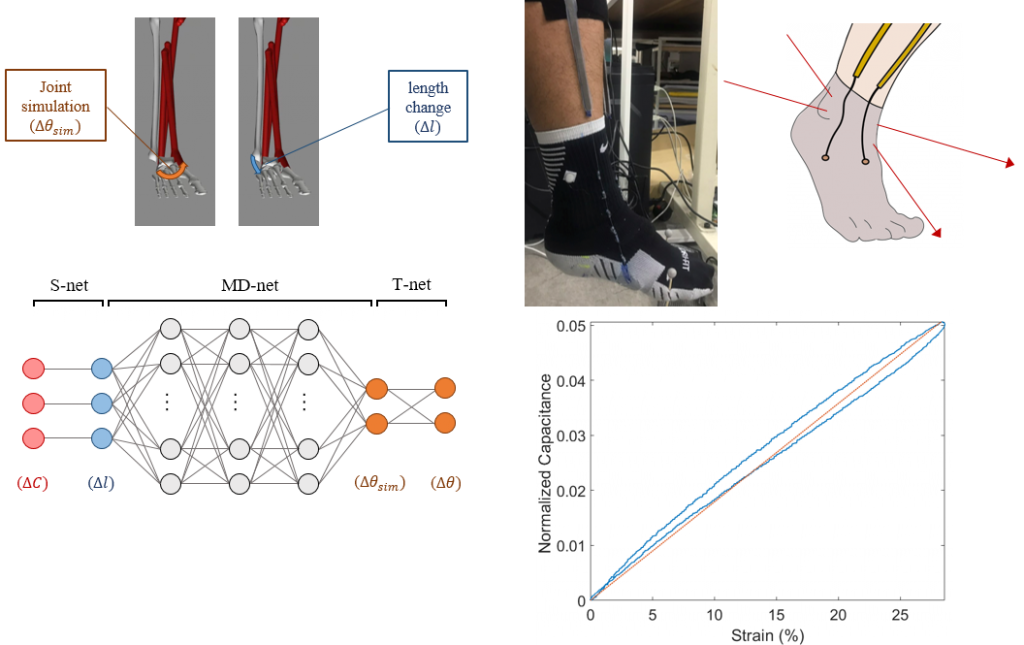
Flexible high-density sEMG (HD-sEMG) sensor with stochastic myo-processor for muscle activation estimation
Surface electromyography (sEMG) signal, which is one of the major neural signals, has been applied to human-machine interface (HMI) for decades. Many studies attempt to achieve accurate estimation and consistent force levels from sEMG signals and applied to control robotic devices. In order to quantitively estimate muscle force, inspired by stochastic myo-processor, using multiple-channel sEMG signals is an effective method to increase signal-to-noise ratio (SNR, i.e. the mean of the amplitude estimation divided by standard deviation). Our research developed a 16-channel flexible high-density sEMG sensor aiming for enhanced human-machine interaction, by providing more information about the muscle activity. In order to finalize design parameters of developed sensor, different prototypes of 1 channel sensors are fabricated to test the effects of the material of electrodes, the size of electrodes, and the internal electrode distance (IED) in terms of SNR and the skin impedance. After the detailed test of 1-channel sensor, the design parameters were selected and expanded to the HD-sEMG sensor. A torque estimation experiment was conducted with the designed HD-sEMG sensor and stochastic myo-processor algorithm. The results indicate the 16-channel HD-sEMG sensor effectively improved the estimation accuracy than using one sEMG sensor. For further application, the proposed HD-sEMG sensor can be used as an enhances human-machine interface to achieve robust control of robotics devices, such as exoskeleton, prosthesis and rehabilitation robot.
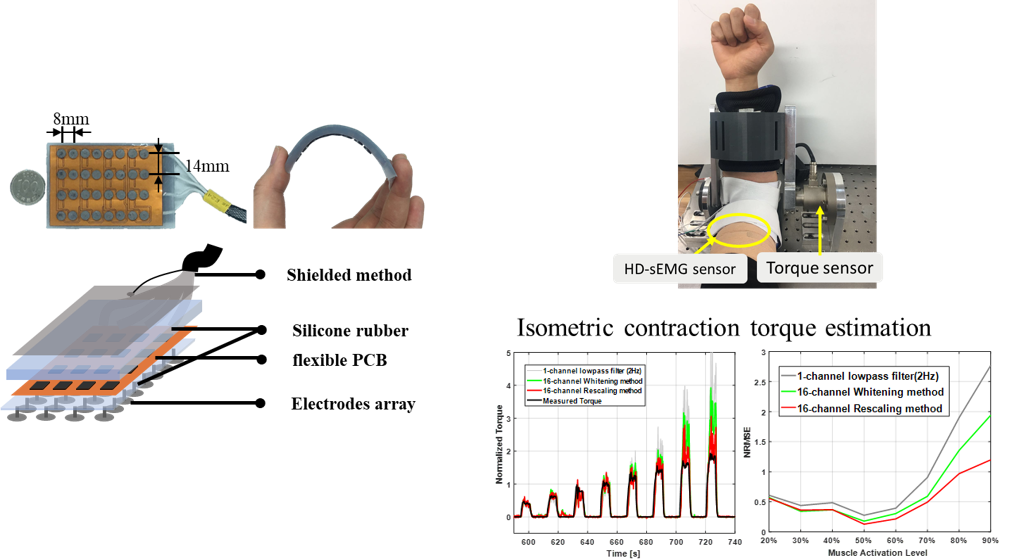